The Hyper-Personalization Trend: Does it Work in Auto Insurance?
Hyper-personalization has taken off. Here are a few modern examples …
- Your streaming channel provides suggestions based on your past viewing behavior.
- Your refrigerator provides recipe suggestions based on what’s available in your fridge.
- Your denim company creates made-to-measure jeans that precisely fit you.
- Your metabolism tracker/flow meter provides diet recommendations based on the carbon dioxide levels you exhale.
What’s the Difference Between Hyper-Personalization and Segmentation?
Hyper-personalization is one-to-one, while segmentation is one-to-many. Idomoo describes the difference this way: “Segmentation is a group photo. It lumps customers by shared traits and demographics. Hyper-personalization is a selfie … providing a unique, Netflix-like ‘just-for-you’ experience.”
With hyper-personalization, companies learn about the individual and then offer the solutions that best cater to each individual’s unique needs.
Data makes this possible. According to Deloitte, the increase of digitization and recent advancements in data technologies have opened the door for personalization. Companies can use machine learning to sift through vast amounts of data in real time, predicting customers’ needs, preferences and future behaviors.
Do Consumers Want Hyper-Personalized Experiences?
While hyper-personalization can be convenient, it can also seem a little creepy. Do individuals really want companies to know so much about them? It seems that the answer is YES, as long as it makes it easier for consumers to get what they want.
According to Forbes, a 2024 State of Customer Service and CX Study from Shep Hyken found that 81% of customers prefer companies that provide a personalized experience. In a different study. McKinsey & Company found that 71% of customers expect personalized interactions, and 76% get frustrated with their interactions aren’t personalized.
Moreover, consumers are willing to trade personal information for personalization, which is a huge win for companies. According to Accenture, consumers are more willing to offer personal data if they receive more personalized pricing, offers and discounts in exchange. In fact, 69% of consumers say they would share significant data on their health, exercise and driving habits if it led to lower prices.
How Could Hyper-Personalization Work in the Insurance Industry?
Hyper-personalization can be used in many industries, including insurance. Here’s a sampling of what’s possible:
- Risk selection. By combining a 360-view of the applicant with policy-level rate adequacy analysis, insurers can provide instant coverage decisions.
- Pricing discounts. Already with usage-based insurance (UBI), drivers allow insurers to monitor their driving habits in exchange for auto insurance discounts. While most UBI discount programs are generalized now, a more personalized pricing model could emerge in the future.
- Policy features and terms. With a more complete understanding of a customer’s risk profile, policies, endorsements and terms can be tailored to better meet each individual’s needs. We’re already seeing tailored policy terms in the parametric insurance space.
- Risk management. With a better understanding of the risk, insurers are able to provide personalized risk management tips, reminders, smart devices and other interventions to reduce claim frequency and severity.
- Claim services. If the insurer has complete information about the claimant’s insured assets, local service providers, geographical nuances and preferences, claim resolution strategies can be initiated faster and deployed more effectively.
How Can You Personalize Auto Insurance Risk Selection?
We’ve known for some time that auto insurance risk selection is far from perfect. Making underwriting decisions on a “group” basis – or by segment – can inevitably lead to some unfair situations and unintended bias.
A common example is the 21-year-old male. In most rate models, young men are charged high rates for insurance no matter how safely they drive, when in fact, 70% of 21-year-old males could be profitable policyholders. The red corvette is yet another classic scenario.
So, how do you separate the rate-adequate from the rate-inadequate?
By taking a more personalized approach to risk selection.
Thanks to machine learning, it’s now possible to calculate drivers’ rate adequacy scores at the policy level. This is better for drivers because they are selected based on their own individual risk attributes (dozens of them) instead of being selected based on a handful of their segment’s characteristics.
It’s also better for insurers. Personalized risk assessment enables insurers to identify and capture the profitable risks within every segment – the risks that are often turned away unnecessarily. With the confidence of individual rate adequacy scoring, insurers can leave all segments turned on while they pursue profitable, uninhibited growth. And, because less labor is required for risk selection processes that are now automated, insurers can scale their businesses without being constrained by a difficult labor market.
See How A More Personalized Approach Can Work for You
With Soteris, auto insurers can affordably deploy personalized risk selection today, in as few as four months. Our technology uses the power of machine learning to score each risk’s rate adequacy, at the policy level, in less than a second. Why be constrained by segments when you can easily make smarter, personalized, one-to-one risk selection decisions?
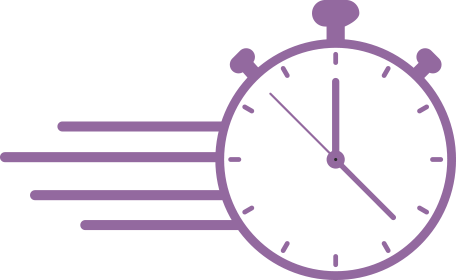