Overcoming Adverse Selection in Auto Insurance
When insurance underwriters don’t have the full picture regarding an applicant’s risk, they are at a disadvantage. This imbalance – in which the insurance applicant has more knowledge than the insurance company – is known as adverse selection. It may result in major losses for insurers. However, new artificial intelligence (AI) capabilities are making it possible to reduce the impact of adverse selection in insurance.
Understanding Adverse Selection in Insurance
Adverse selection occurs when one party knows more than the other in a transaction. For example, imagine someone is buying a vase at an estate sale. The buyer knows the vase is a rare antique worth millions. The seller does not know this. Clearly, the buyer has an extreme advantage and will be able to negotiate a much better deal than would be possible if the seller knew everything the buyer did.
In other situations, adverse selection may benefit the seller – for example, if someone selling a house knows that the neighborhood is plagued by noise and crime at night, but the buyer does not know this.
Adverse selection is frequently a problem in insurance, often benefiting the policyholder. Before writing a policy, insurers try to gather as much information as possible. They ask questions about car usage and location. They also review past insurance claims and look at the applicant’s DMV records for signs of risky driving. Nevertheless, the insurance applicant will always know more about his or her driving habits than the insurance company.
For example, imagine a driver has a bad habit of texting while driving. Despite some close calls, the driver has never been in a crash or received a ticket for distracted driving. As a result, the insurer has no indication that this is a problem and therefore provides a policy at a low rate. Then, the driver causes a serious crash with significant property damage and injuries – and the insurer is on the hook for the losses.
Many Insurance Applicants Lie
In auto insurance, application fraud is a common source of adverse risk selection.
According to a ValuePenguin survey, Millennials are particularly likely to lie to their insurers to save money, with 39% admitting to deception compared to 16% of Gen Xers and 3% of Baby Boomers:
16% have used a different address to get a better rate.
15% have said they use their vehicle less than they actually do.
15% have failed to report all the people who drive their car.
These omissions clearly give drivers an unfair advantage when it comes to underwriting. For example, an underwriter might approve a policy based on the applicant’s driving history, not knowing that the applicant’s boyfriend – who has a history of DUIs and crashes – will be driving the car to work every day.
How AI Can Level the Playing Field
Insurers are frequently at a disadvantage because they lack information the applicant possesses. This may be due to a lack of disclosure – for example, when an applicant does not volunteer information about risky driving habits. It may also be the result of insurance application fraud, such as when applicants lie about their garaging address or the way they use their car. Either way, rates may not reflect true risk, which may lead to losses for insurers.
AI can level the playing field by combing through data to spot red flags, discrepancies and potential omissions, giving insurers a more informed understanding of the risk they’re considering. With the right software, an algorithm can alert the underwriter of the potential for rate-inadequacy, and the need for further review, when discrepancies and omissions are identified.
AI makes it possible for auto insurers to know better and perform better.
Get to Know Soteris
Soteris uses the power of machine learning to score the rate adequacy of each individual auto insurance risk in less than one second.
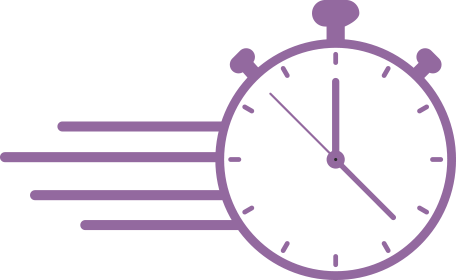